YaRN: New Approach to Expanding Context in LLaMa-2 Up to 128k Tokens


In Brief
YaRN, a new method for expanding context in language models, uses the RoPE technique for positional coding to accommodate large contexts.
It incorporates a temperature parameter and is adaptable to existing models like Hugging Face.
Although it requires retraining on data containing extended contexts, YaRN offers valuable insights and improved performance in various natural language processing tasks.
A new method known as YaRN (Yet Another RoPE for Transformers) has emerged, offering the potential to extend context capabilities in large language models (LLMs) using the RoPE technique for positional coding. This approach, as detailed in a recent article, provides the means to expand context up to 64k or even 128k tokens. This innovation is particularly notable as it addresses the growing demand for models that can accommodate substantial context, such as extended texts or lengthy message histories.
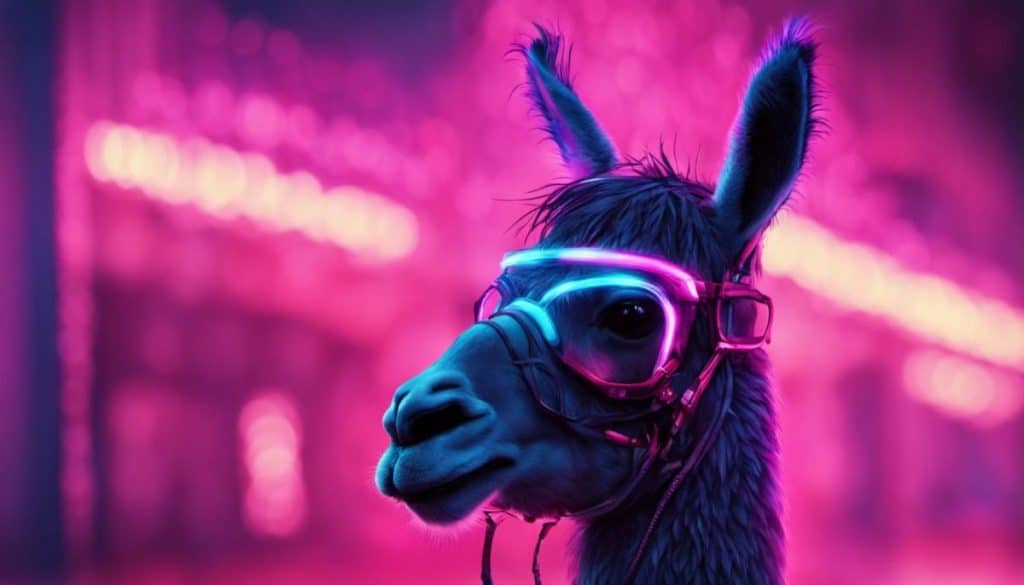
The RoPE method involves rotating vectors in space at specific angles based on their positions, and is particularly used in models like LLaMa-2. The YaRN method differs from earlier modifications, though, by adding a brand-new component: a temperature parameter that is crucial in affecting how quickly people pay attention after the softmax operation. This integration of temperature control is significant because it keeps the attention mechanisms’ original structure and prevents the need for significant changes to the existing codebase.
An intriguing aspect of YaRN’s implementation is its adaptability with existing models hosted on platforms like Hugging Face. By harnessing the power of these readily available models, researchers and practitioners can experiment with and explore the YaRN method with relative ease.
Size | Context | Link |
---|---|---|
7B | 64K | NousResearch/Yarn-Llama-2-7b-64k |
7B | 128K | NousResearch/Yarn-Llama-2-7b-128k |
13B | 64K | NousResearch/Yarn-Llama-2-13b-64k |
13B | 128K | NousResearch/Yarn-Llama-2-13b-128k |
It is worth noting that YaRN, like other novel techniques, requires retraining on data containing extended contexts, albeit in a modest quantity—approximately 0.1% of the pretraining data. The primary consideration moving forward pertains to the computational resources necessary for efficiently inferring with these expanded-context models, an aspect that will play a pivotal role in the practical implementation of this innovative approach.
- YaRN opens the door to more extensive contextual understanding, offering applications that span various domains, from literature analysis to conversational AI. As the AI community continues to explore methods for enhancing model capabilities, YaRN’s nuanced approach to extending context holds the potential to provide valuable insights and improved performance in various natural language processing tasks.
- In July, Meta has released LLaMa-2-Chat models, a game-changing open-source language model with 70 billion parameters, comparable to and outperforming GPT-3.5 on certain benchmarks. The model is commercially friendly, pretrained on 2T tokens, and has strong MMLU scores. It is the first model of its size fine-tuned using RLHF, making it completely free for commercial use. LLaMa-2-Chat showcases exceptional performance on mathematical problems and is available in various sizes.
Read more about AI:
Disclaimer
In line with the Trust Project guidelines, please note that the information provided on this page is not intended to be and should not be interpreted as legal, tax, investment, financial, or any other form of advice. It is important to only invest what you can afford to lose and to seek independent financial advice if you have any doubts. For further information, we suggest referring to the terms and conditions as well as the help and support pages provided by the issuer or advertiser. MetaversePost is committed to accurate, unbiased reporting, but market conditions are subject to change without notice.
About The Author
Damir is the team leader, product manager, and editor at Metaverse Post, covering topics such as AI/ML, AGI, LLMs, Metaverse, and Web3-related fields. His articles attract a massive audience of over a million users every month. He appears to be an expert with 10 years of experience in SEO and digital marketing. Damir has been mentioned in Mashable, Wired, Cointelegraph, The New Yorker, Inside.com, Entrepreneur, BeInCrypto, and other publications. He travels between the UAE, Turkey, Russia, and the CIS as a digital nomad. Damir earned a bachelor's degree in physics, which he believes has given him the critical thinking skills needed to be successful in the ever-changing landscape of the internet.
More articles

Damir is the team leader, product manager, and editor at Metaverse Post, covering topics such as AI/ML, AGI, LLMs, Metaverse, and Web3-related fields. His articles attract a massive audience of over a million users every month. He appears to be an expert with 10 years of experience in SEO and digital marketing. Damir has been mentioned in Mashable, Wired, Cointelegraph, The New Yorker, Inside.com, Entrepreneur, BeInCrypto, and other publications. He travels between the UAE, Turkey, Russia, and the CIS as a digital nomad. Damir earned a bachelor's degree in physics, which he believes has given him the critical thinking skills needed to be successful in the ever-changing landscape of the internet.