Google’s Analysis Reveals Surprising Insights into LLMs and Search Engine Accuracy


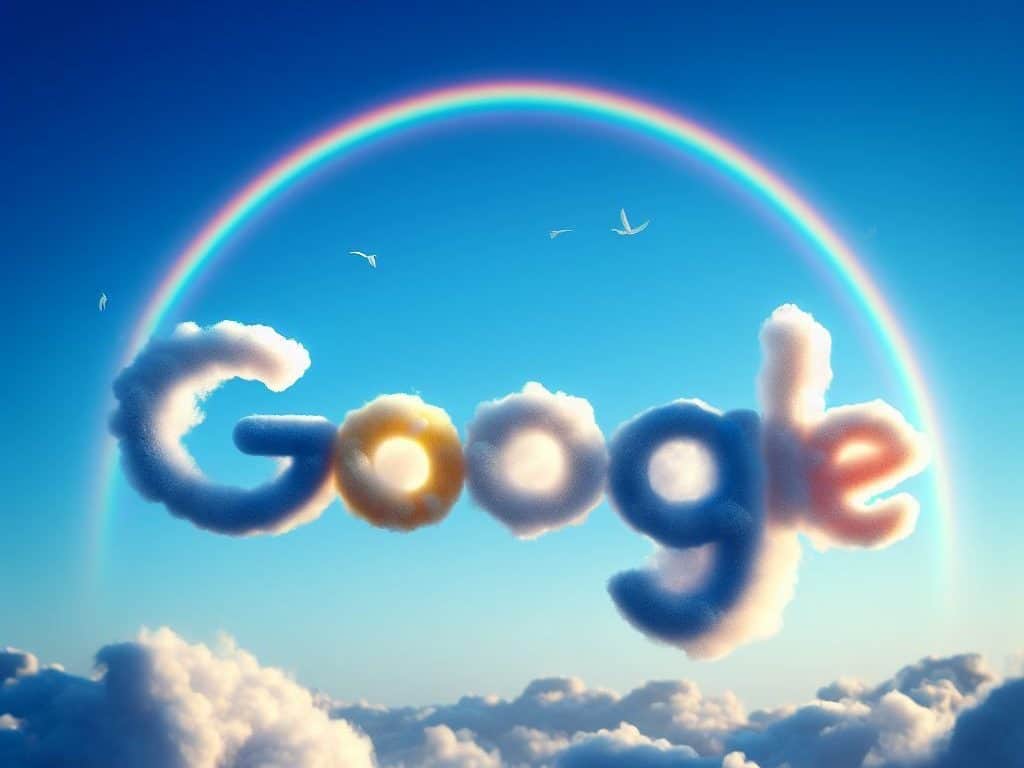
In early September, Yandex hosted a private mini-conference on generative AI, providing a platform for deep dives into the world of AI. Nevertheless, the conference brought forth significant revelations, especially concerning the much-anticipated YandexGPT 2.
Yandex’s unveiling of YandexGPT 2 had the AI community buzzing with anticipation. The creators of this model explored various distinguishing features, including a specialized module designed to search and provide answers based on search result data.
Notably, the team’s revelations unveiled a striking aspect: even when trained on a vast repository of internal Yandex data spanning over a decade of work on neural search mechanisms, this proprietary model still fell short of the formidable GPT-4. This significant development, underscores the remarkable strides achieved by GPT-4. This observation accentuates GPT-4’s supremacy over both proprietary developments and prior open-source iterations.
Expanding on such foundational insights, Google did a study to assess the accuracy of responses from Large Language Models (LLMs) empowered with search engine access. Although the notion of integrating an external tool with LLMs is not novel, Google found that the complexity lies in the nuanced assessment and validation of these models. Crucial factors shaping this integration encompass the selection of a carefully crafted prompt and the intrinsic capabilities of the LLMs.
Google’s LLM Test Methodology
A curated corpus of 600 questions was divided into four distinct groups. Each group prioritized factual accuracy, but one group stood out for its inclusion of questions rooted in false premises.
For instance, questions like “what did Trump write after being unbanned on Twitter?” contained an inaccurate premise, as Trump had not been unbanned. The remaining three groups introduced variables of answer obsolescence: never, rarely and often. In the “never” group, LLMs were expected to answer purely from memory, whereas questions about recent events required a real-time search. Each group consisted of 125 questions.
The questions were presented to a diverse range of models. Intriguingly, questions containing false premises revealed the dominance of GPT-4 and ChatGPT, which adeptly refuted such premises, indicating their specific training to handle such challenges.
A comparative analysis ensued, pitting ChatGPT, GPT-4, Google search (based on text snippets or first-page answers), and PPLX.AI (a platform leveraging ChatGPT to aggregate Google’s responses, aimed at developers) against each other. In this context, LLMs provided answers exclusively from their memory.
In a noteworthy observation, Google search provided correct answers in 40% of cases on average across the four groups. The accuracy for “eternal” questions stood at 70%, while false-premise questions plummeted to just 11%. ChatGPT’s performance clocked in at 26% on average, while GPT-4 reached 28%, impressively responding to false-premise questions in 42% of instances. PPLX.AI demonstrated a 52% success rate.
The study delved deeper by integrating a novel approach. Each question prompted a Google search, with the results incorporated into the prompt. LLMs were then required to “read” this information before composing their answers. This technique allowed for Few-Shot learning (where examples are presented in the prompt to guide the model) and thoughtful step-by-step consideration before answering.
The results were nothing short of fascinating. GPT-4 exhibited a remarkable 77% quality rating, answering “eternal” questions with 96% accuracy and addressing false-premise questions with a commendable 75% precision. While ChatGPT offered slightly less impressive metrics, it outperformed both PPLX.AI and Google search.
Mastering AI Prompt Design: Key Insights from PPLX.AI and Google Experts
The ability to guide Large Language Models (LLMs) effectively through a labyrinth of information is no small feat. However, a recent exploration of AI prompts has illuminated key strategies that promise to enhance the quality of LLM-generated responses, offering a glimpse into the nuanced mechanics of AI assistance.
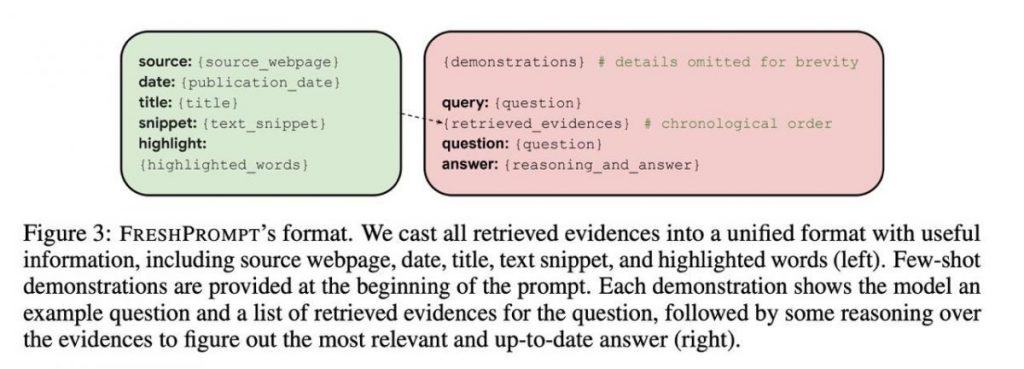
The foundation for this revelation was established through careful prompt structuring. This method consists of multiple components, offering a clear path to achieving precise answers, firmly grounded in contextual comprehension. The initial aspect includes illustrative examples, serving as guiding markers, directing LLMs toward the correct answer based on contextual clues.
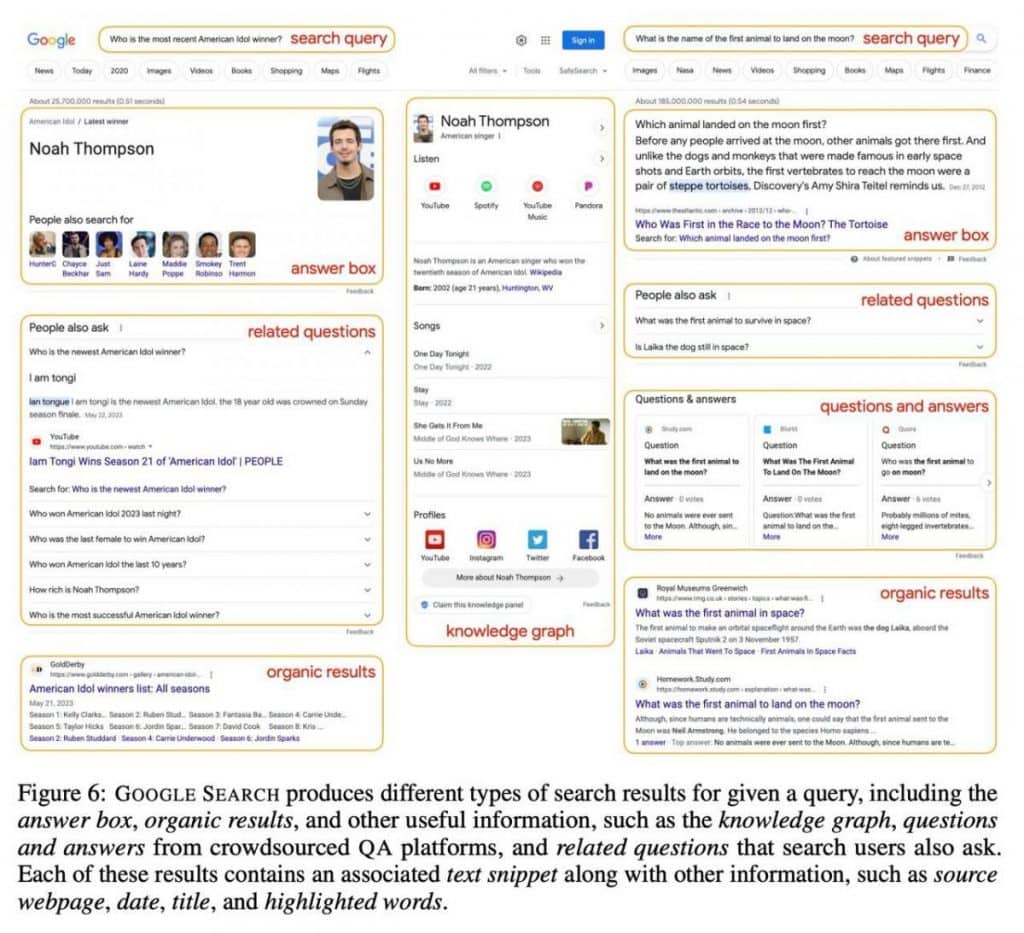
the second layer reveals the actual query along with 10-15 search results. These results go beyond mere web page links, encompassing a wealth of information, including textual content, relevant queries, questions, answers, and knowledge graphs. This approach equips the AI with a comprehensive knowledge library.
The sophistication of this system goes further. A crucial discovery emerged when arranging the links chronologically within the prompt, placing the most recent additions at the end. This chronological arrangement mirrors the evolving nature of information, enabling the model to discern the timeline of changes. The inclusion of dates in each example played a pivotal role in enhancing contextual understanding.
While the code to employ this nuanced prompt structuring is eagerly anticipated, its absence has prompted enthusiasts to venture into rewriting prompt templates based on images provided.
Several key takeaways emerge from this foray into the mechanics of AI prompts:
1) PPLX.AI, a platform that leverages ChatGPT to aggregate Google’s responses, has emerged as a promising option. Even Google employees have hinted at its superiority.
2) Experimentation with various elements yielded enhancements in response metrics. Precision in prompt construction, it seems, is an art unto itself.
3) GPT-4 demonstrates commendable proficiency in processing extensive sets of news and texts. While it may not be characterized as “excellent,” its quality even in rapidly changing news scenarios hovers around the 60% mark. The AI community is encouraged to evaluate such metrics critically.
4) As the AI ecosystem continues to expand, LLMs integrated into search engines are poised to become ubiquitous, catering to a broad spectrum of users. The presence of AI in everyday search experiences is on an upward trajectory, signifying a transformative shift in the way information is accessed and processed.
The multifaceted approach offers a promising way to get accurate answers from these sophisticated language models because it includes illustrative examples, a clearly defined query, and a wealth of contextual information. The chronological arrangement of the links within the prompts led to a significant insight, underscoring the significance of adapting to the dynamic nature of information. LLMs can navigate the timeline of changes thanks to this temporal awareness, which improves their contextual comprehension.
Disclaimer
In line with the Trust Project guidelines, please note that the information provided on this page is not intended to be and should not be interpreted as legal, tax, investment, financial, or any other form of advice. It is important to only invest what you can afford to lose and to seek independent financial advice if you have any doubts. For further information, we suggest referring to the terms and conditions as well as the help and support pages provided by the issuer or advertiser. MetaversePost is committed to accurate, unbiased reporting, but market conditions are subject to change without notice.
About The Author
Damir is the team leader, product manager, and editor at Metaverse Post, covering topics such as AI/ML, AGI, LLMs, Metaverse, and Web3-related fields. His articles attract a massive audience of over a million users every month. He appears to be an expert with 10 years of experience in SEO and digital marketing. Damir has been mentioned in Mashable, Wired, Cointelegraph, The New Yorker, Inside.com, Entrepreneur, BeInCrypto, and other publications. He travels between the UAE, Turkey, Russia, and the CIS as a digital nomad. Damir earned a bachelor's degree in physics, which he believes has given him the critical thinking skills needed to be successful in the ever-changing landscape of the internet.
More articles

Damir is the team leader, product manager, and editor at Metaverse Post, covering topics such as AI/ML, AGI, LLMs, Metaverse, and Web3-related fields. His articles attract a massive audience of over a million users every month. He appears to be an expert with 10 years of experience in SEO and digital marketing. Damir has been mentioned in Mashable, Wired, Cointelegraph, The New Yorker, Inside.com, Entrepreneur, BeInCrypto, and other publications. He travels between the UAE, Turkey, Russia, and the CIS as a digital nomad. Damir earned a bachelor's degree in physics, which he believes has given him the critical thinking skills needed to be successful in the ever-changing landscape of the internet.