Today’s Large Language Models Will Be Small Models, According to a Researcher at OpenAI


Hyung Won Chung, an accomplished AI researcher who was formerly employed by Google Brain and is currently a member of the OpenAI team, gave a thought-provoking 45-minute speech in which he explored the world of large language models in 2023. Chung has experience in the field; he was the first author of the Google paper “Scaling Instruction-Finetuned Language Models,” which examines how large language models can be trained to follow instructions.
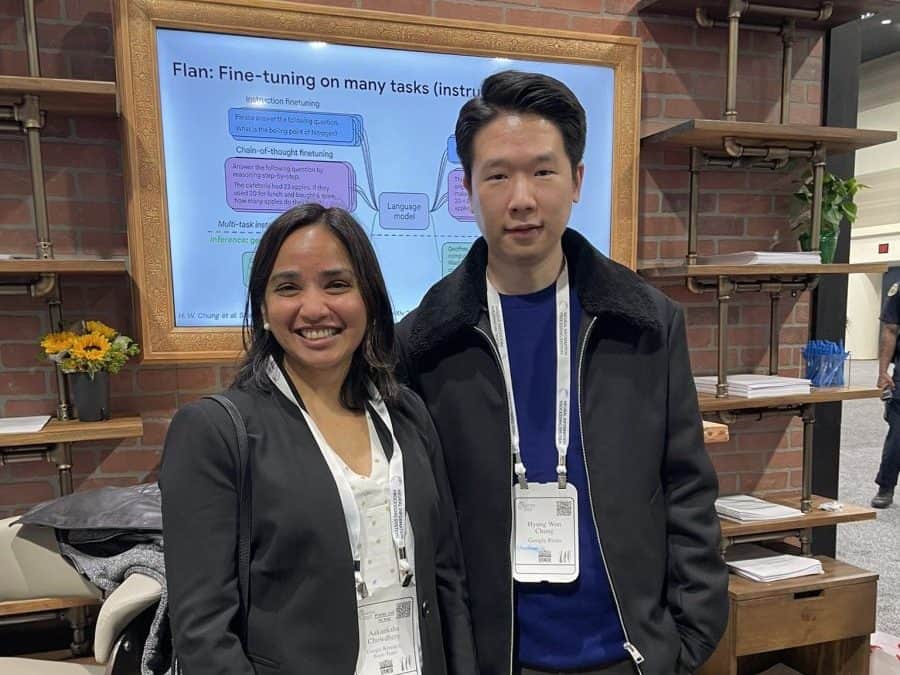
Chung emphasises the world of extensive language models as being dynamic. In the world of LLMs, the guiding principle is constantly evolving, in contrast to traditional fields where fundamental assumptions typically remain stable. With the upcoming generation of models, what is currently thought to be impossible or impractical may become possible. He emphasises the significance of prefacing most claims about LLM capabilities with “for now”. A model can perform a task; it just hasn’t done so yet.
Large models of today will be small models in only a few years
Hyung Won Chung, OpenAI
The need for meticulous documentation and reproducibility in AI research is one of the most important lessons to be learned from Chung’s speech. It’s crucial to thoroughly document ongoing work as the field develops. This strategy guarantees that experiments can be quickly replicated and revisited, enabling researchers to build on earlier work. Through this practise, it is acknowledged that capabilities may develop in the future that weren’t practical during the initial research.
Chung dedicates a portion of his talk to elucidating the intricacies of data and model parallelism. For those interested in delving deeper into the technical aspects of AI, this section provides valuable insights into the inner workings of these parallelism techniques. Understanding these mechanisms is crucial for optimizing large-scale model training.
Chung posits that the current objective function, Maximum Likelihood, used for LLM pre-training is a bottleneck when it comes to achieving truly massive scales, such as 10,000 times the capacity of GPT-4. As machine learning progresses, manually designed loss functions become increasingly limiting.
Chung suggests that the next paradigm in AI development involves learning functions through separate algorithms. This approach, although in its infancy, holds the promise of scalability beyond current constraints. He also highlights ongoing efforts, such as Reinforcement Learning from Human Feedback (RLHF) with Rule Modeling, as steps in this direction, although challenges remain to be overcome.
Disclaimer
In line with the Trust Project guidelines, please note that the information provided on this page is not intended to be and should not be interpreted as legal, tax, investment, financial, or any other form of advice. It is important to only invest what you can afford to lose and to seek independent financial advice if you have any doubts. For further information, we suggest referring to the terms and conditions as well as the help and support pages provided by the issuer or advertiser. MetaversePost is committed to accurate, unbiased reporting, but market conditions are subject to change without notice.
About The Author
Damir is the team leader, product manager, and editor at Metaverse Post, covering topics such as AI/ML, AGI, LLMs, Metaverse, and Web3-related fields. His articles attract a massive audience of over a million users every month. He appears to be an expert with 10 years of experience in SEO and digital marketing. Damir has been mentioned in Mashable, Wired, Cointelegraph, The New Yorker, Inside.com, Entrepreneur, BeInCrypto, and other publications. He travels between the UAE, Turkey, Russia, and the CIS as a digital nomad. Damir earned a bachelor's degree in physics, which he believes has given him the critical thinking skills needed to be successful in the ever-changing landscape of the internet.
More articles

Damir is the team leader, product manager, and editor at Metaverse Post, covering topics such as AI/ML, AGI, LLMs, Metaverse, and Web3-related fields. His articles attract a massive audience of over a million users every month. He appears to be an expert with 10 years of experience in SEO and digital marketing. Damir has been mentioned in Mashable, Wired, Cointelegraph, The New Yorker, Inside.com, Entrepreneur, BeInCrypto, and other publications. He travels between the UAE, Turkey, Russia, and the CIS as a digital nomad. Damir earned a bachelor's degree in physics, which he believes has given him the critical thinking skills needed to be successful in the ever-changing landscape of the internet.