Text-to-SVG: Berkeley announced abstracting pixel-based diffusion model

In Brief
Text-to-image synthesis – UC Berkeley researchers demonstrate vector graphics with text-conditioned diffusion models
In text-to-image synthesis, diffusion models have demonstrated outstanding outcomes. Diffusion models learn to produce raster images of extremely diverse objects and situations using enormous databases of annotated pics. However, for digital icons, graphics, and stickers, designers typically employ vector representations of images like Scalable Vector Graphics (SVGs). Vector graphics are small and may be scaled to any size.
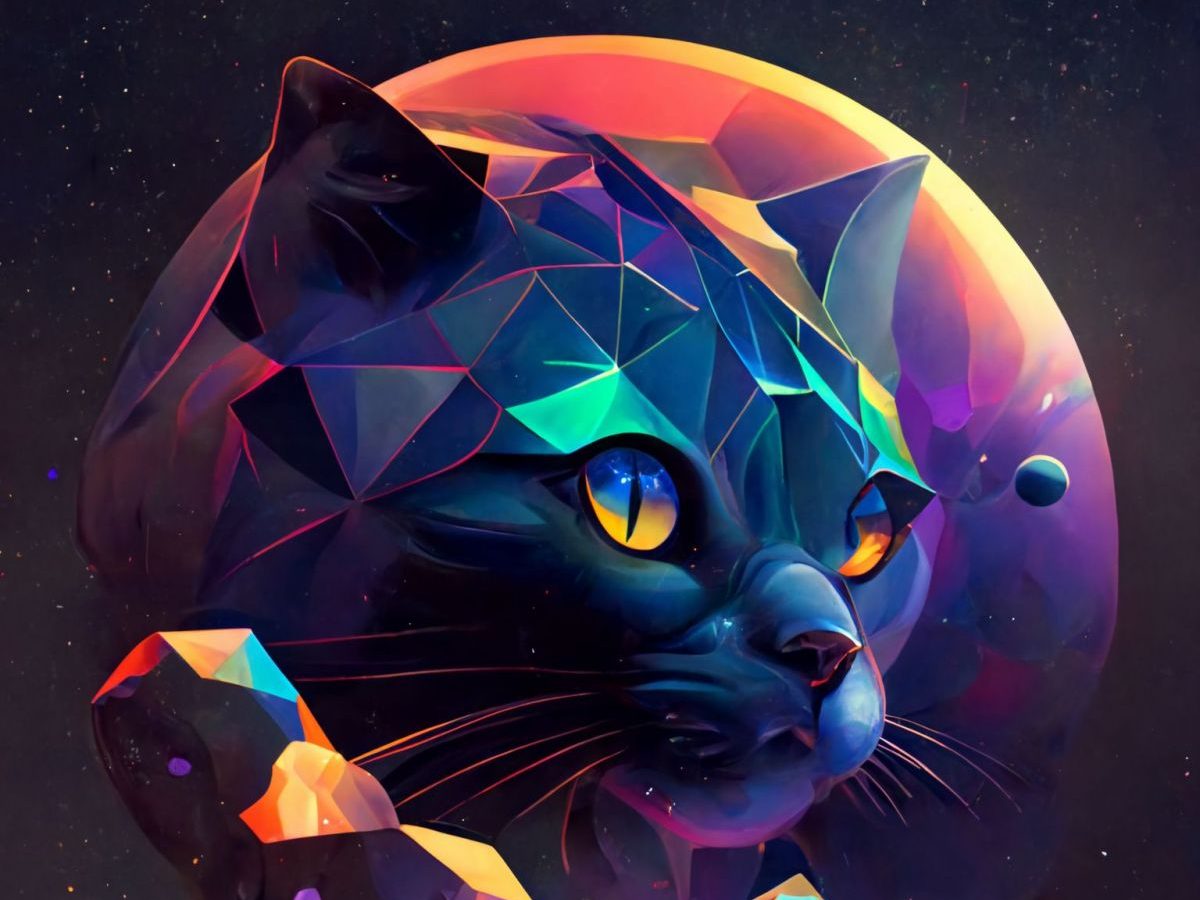
UC Berkeley demonstrates how to produce vector graphics that can be exported as SVG using a text-conditioned diffusion model that was trained on picture pixel representations. It accomplishes this without using extensive collections of SVGs with captions. Instead, Berkeley researchers vectorize a text-to-image diffusion sample and fine-tune it with a Score Distillation Sampling loss, motivated by recent work on text-to-3D synthesis.
Example generated vectors
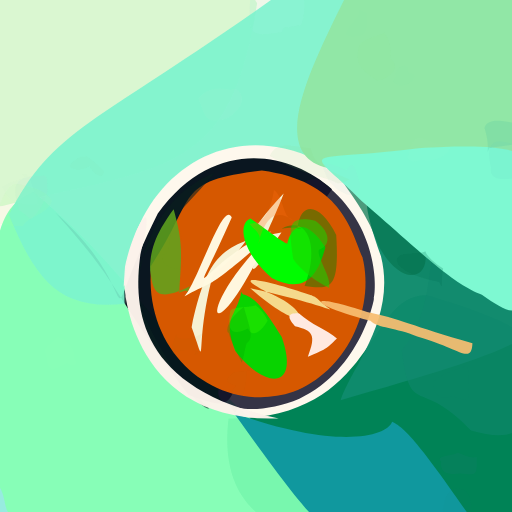
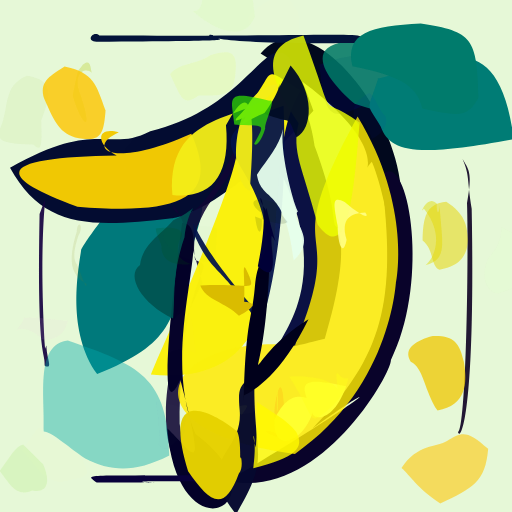
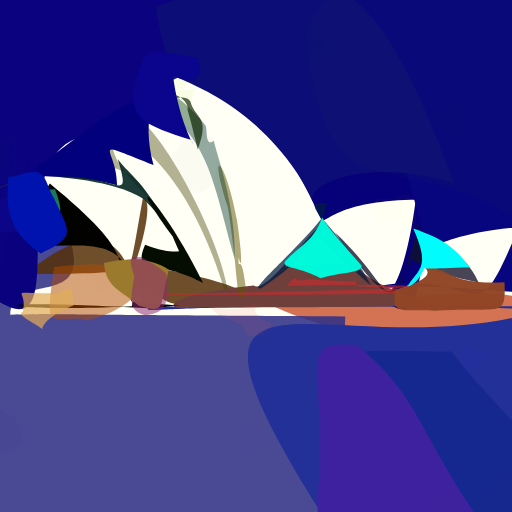
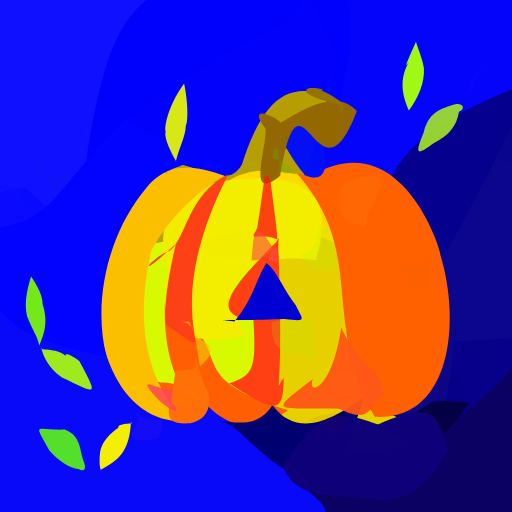
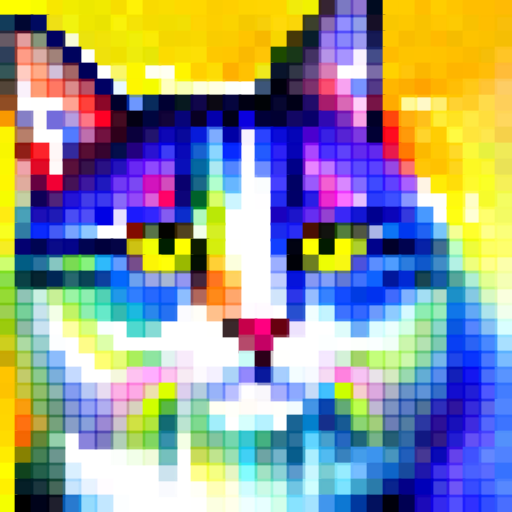
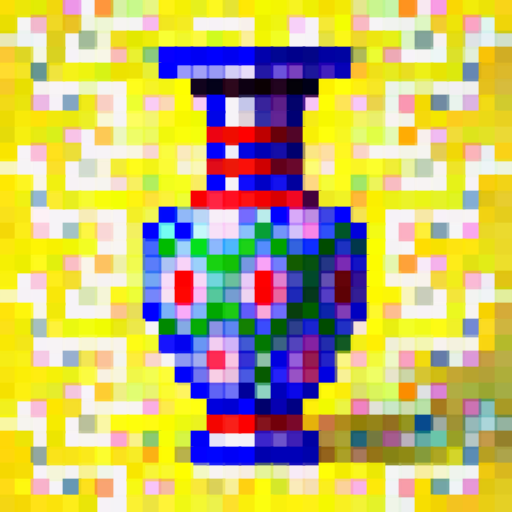
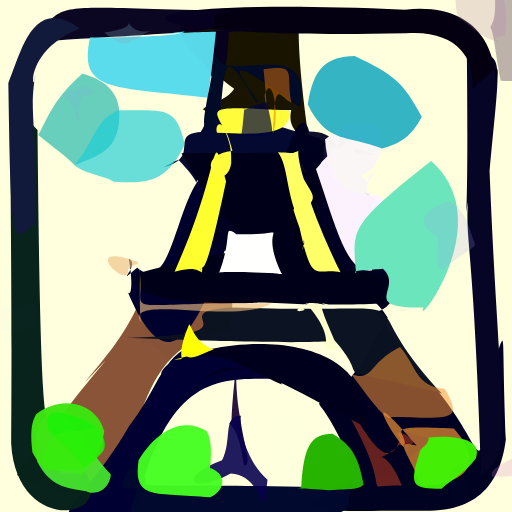
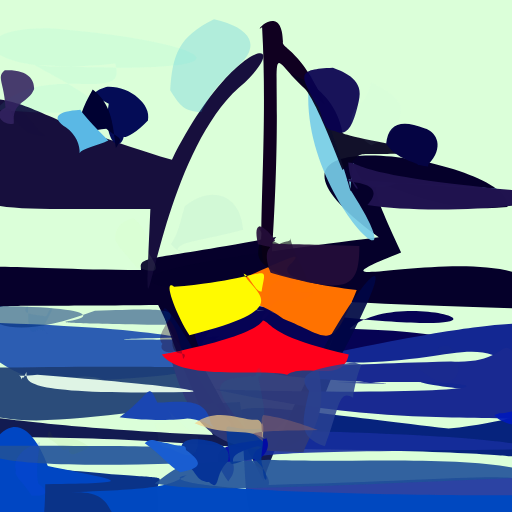
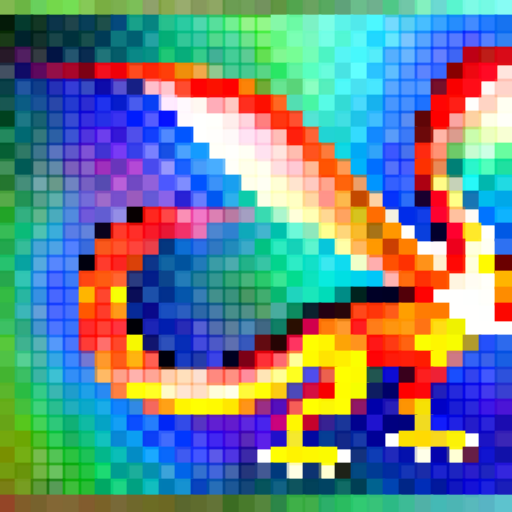
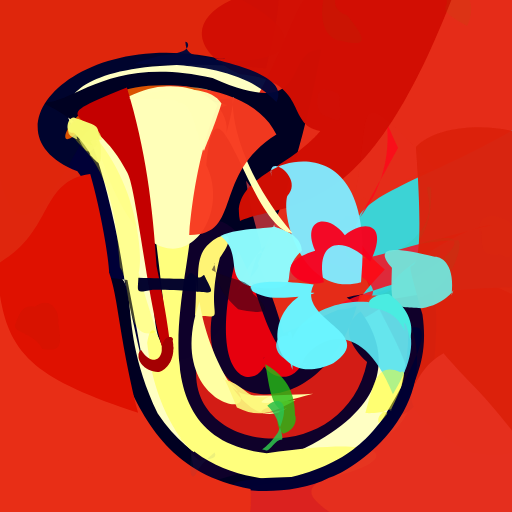
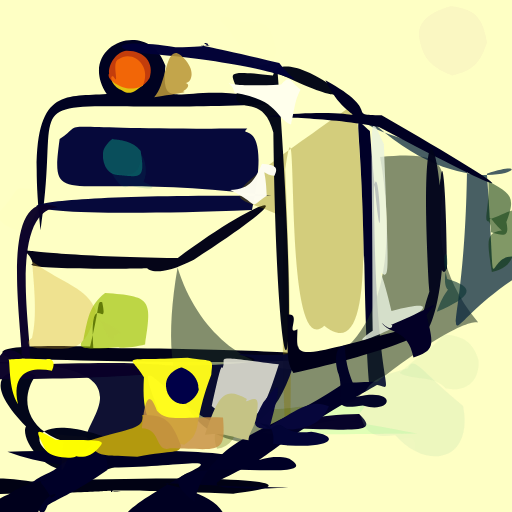
Check out the freshly generated SVG gallery here.
Vector graphics are small but maintain their sharpness when scaled to any size. Researchers at Berkeley improve an image-text loss based on Score Distillation Sampling to optimize vector graphics. The DiffVG differentiable SVG renderer, which is used by VectorFusion, makes inverse visuals possible.
Additionally, VectorFusion allows a multi-stage configuration that is more effective and of higher quality. This method starts by taking raster samples from the text-to-image diffusion model called Stable Diffusion. The samples are then automatically traced by VectorFusion using LIVE. These samples, nevertheless, frequently lack detail, are boring, or are difficult to adapt to vector graphics. Enhancing vibrancy and textual consistency through Score Distillation Sampling.
VectorFusion can produce pixel art in the style of old video games by limiting SVG paths to squares on a grid.
This approach is easily expanded to support text-to-sketch generation. In order to learn an abstract line drawing that accurately represents the user-supplied text, we first draw 16 randomly chosen strokes. Then, we optimize our latent Score Distillation Sampling loss.
Read related articles:
Disclaimer
In line with the Trust Project guidelines, please note that the information provided on this page is not intended to be and should not be interpreted as legal, tax, investment, financial, or any other form of advice. It is important to only invest what you can afford to lose and to seek independent financial advice if you have any doubts. For further information, we suggest referring to the terms and conditions as well as the help and support pages provided by the issuer or advertiser. MetaversePost is committed to accurate, unbiased reporting, but market conditions are subject to change without notice.
About The Author
Damir is the team leader, product manager, and editor at Metaverse Post, covering topics such as AI/ML, AGI, LLMs, Metaverse, and Web3-related fields. His articles attract a massive audience of over a million users every month. He appears to be an expert with 10 years of experience in SEO and digital marketing. Damir has been mentioned in Mashable, Wired, Cointelegraph, The New Yorker, Inside.com, Entrepreneur, BeInCrypto, and other publications. He travels between the UAE, Turkey, Russia, and the CIS as a digital nomad. Damir earned a bachelor's degree in physics, which he believes has given him the critical thinking skills needed to be successful in the ever-changing landscape of the internet.
More articles

Damir is the team leader, product manager, and editor at Metaverse Post, covering topics such as AI/ML, AGI, LLMs, Metaverse, and Web3-related fields. His articles attract a massive audience of over a million users every month. He appears to be an expert with 10 years of experience in SEO and digital marketing. Damir has been mentioned in Mashable, Wired, Cointelegraph, The New Yorker, Inside.com, Entrepreneur, BeInCrypto, and other publications. He travels between the UAE, Turkey, Russia, and the CIS as a digital nomad. Damir earned a bachelor's degree in physics, which he believes has given him the critical thinking skills needed to be successful in the ever-changing landscape of the internet.