Facebook Develops a New Method for Doubling the Performance of AI Transformers

In Brief
Facebook has developed a new method for doubling the performance of AI transformers based on the transformer architecture.
The new method finds the most similar patches in the gaps between processing different blocks and combines them to reduce computational complexity.
Facebook has developed a new method for doubling the performance of AI transformers. The method is based on the transformer architecture and is specifically designed for long-form text such as books, articles, and blogs. The goal of the new AI transformer is to improve the performance of transformer-based models on long-form text by making them more efficient and effective at handling long sequences. The results of the AI transformer are very promising, and this new method has a chance to help improve the performance of transformer-based models on a variety of tasks.
This new method is expected to have a significant impact on natural language processing tasks, such as language translation, summarization, and question-answering systems. It is also expected to lead to the development of more sophisticated AI models that can handle longer and more complex texts.
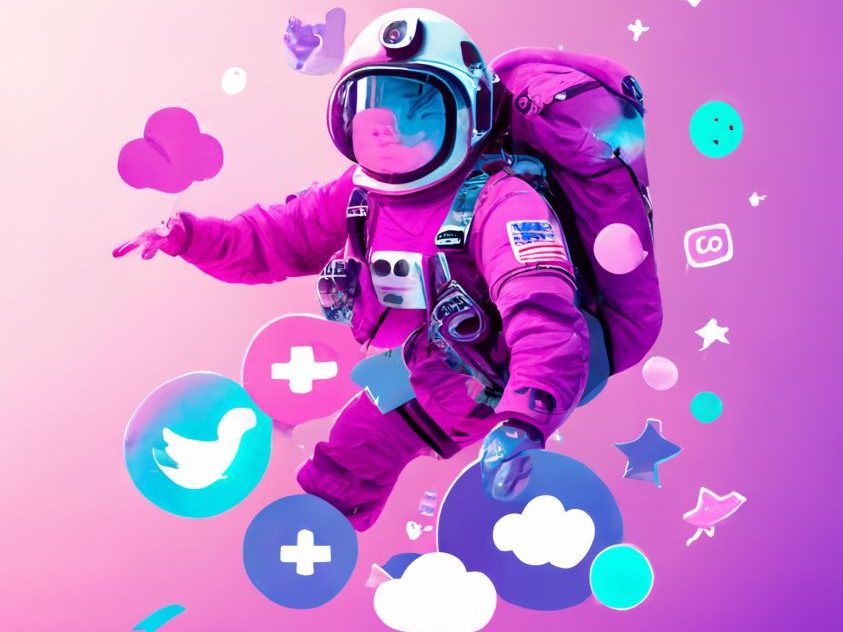
To process the image, modern transformers cut it into patches (usually squares: see the gif below) and then operate on representations of these particles, each of which is represented by a “token.” Transformers, as we know, work slower the more of these token pieces there are (this applies to both texts and images), and the most common transformer has a quadratic relationship. That is, as more tokens are added, the slower the processing becomes. To address this issue, researchers have proposed various techniques to reduce the number of tokens required for image processing, such as hierarchical and adaptive pooling. These methods aim to maintain the quality of the output while minimizing the computational cost.
The new method finds the most similar patches in the gaps between processing different blocks and combine them to reduce computational complexity. The share of merged tokens is a hyperparameter; the higher it is, the lower the quality but also the higher the acceleration. Experiments show that it is possible to merge approximately 40% of tokens with a quality loss of 0.1-0.4% and get double acceleration (thus consiming less memory). This new method is a promising solution for reducing the computational complexity of image processing and could allow for faster and more efficient processing without compromising the quality of the final output.
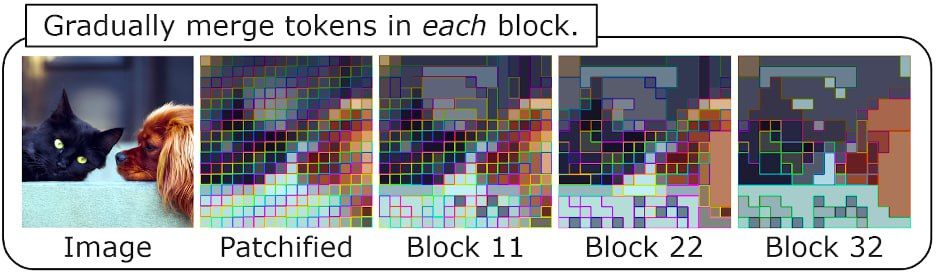
Such engineering approaches based on ingenuity and understanding how something works look very appealing. Also, Meta’s developers promise to bring more to StableDiffusion in order to speed things up there as well. It’s awesome that, because transformers are everywhere, such tricks can be quickly implemented in a wide range of models. This shows the potential for engineering solutions to have a broad impact across various industries. It will be interesting to see how these advancements in transformer models will continue to evolve and improve over time.
- Meta AI and Paperswithcode have released the first 120B model Galactica trained on scientific texts, allowing for more accurate and faster predictions. The goal of Galactica is to help researchers separate the important from the irrelevant.
Read more related news:
Disclaimer
In line with the Trust Project guidelines, please note that the information provided on this page is not intended to be and should not be interpreted as legal, tax, investment, financial, or any other form of advice. It is important to only invest what you can afford to lose and to seek independent financial advice if you have any doubts. For further information, we suggest referring to the terms and conditions as well as the help and support pages provided by the issuer or advertiser. MetaversePost is committed to accurate, unbiased reporting, but market conditions are subject to change without notice.
About The Author
Damir is the team leader, product manager, and editor at Metaverse Post, covering topics such as AI/ML, AGI, LLMs, Metaverse, and Web3-related fields. His articles attract a massive audience of over a million users every month. He appears to be an expert with 10 years of experience in SEO and digital marketing. Damir has been mentioned in Mashable, Wired, Cointelegraph, The New Yorker, Inside.com, Entrepreneur, BeInCrypto, and other publications. He travels between the UAE, Turkey, Russia, and the CIS as a digital nomad. Damir earned a bachelor's degree in physics, which he believes has given him the critical thinking skills needed to be successful in the ever-changing landscape of the internet.
More articles

Damir is the team leader, product manager, and editor at Metaverse Post, covering topics such as AI/ML, AGI, LLMs, Metaverse, and Web3-related fields. His articles attract a massive audience of over a million users every month. He appears to be an expert with 10 years of experience in SEO and digital marketing. Damir has been mentioned in Mashable, Wired, Cointelegraph, The New Yorker, Inside.com, Entrepreneur, BeInCrypto, and other publications. He travels between the UAE, Turkey, Russia, and the CIS as a digital nomad. Damir earned a bachelor's degree in physics, which he believes has given him the critical thinking skills needed to be successful in the ever-changing landscape of the internet.