XLM-V: A New Method of Multilingual Masked Language Models That Attempts to Address the Problem of Vocabulary Bottleneck

In Brief
The article raises the following problem: language models increase in parameters, grow in depth, but the vocabulary is still the same in size.
Researchers start training a new model with 1 million tokens from the vocabulary in an unexpected way.
The researchers were determined to see what kind of improvement they could make with such a significant increase in tokens.
The issue raised by the article entitled “XLM-V: Overcoming the Vocabulary Bottleneck in Multilingual Masked Language Models” is that when language models’ parameters and depth increase, their vocabulary sizes remain unchanged. For instance, the mT5 model has 13B parameters but a 250K-word vocabulary that supports more than 100 languages. Thus, each language has approximately 2,500 unique tokens, which is obviously a very small number.
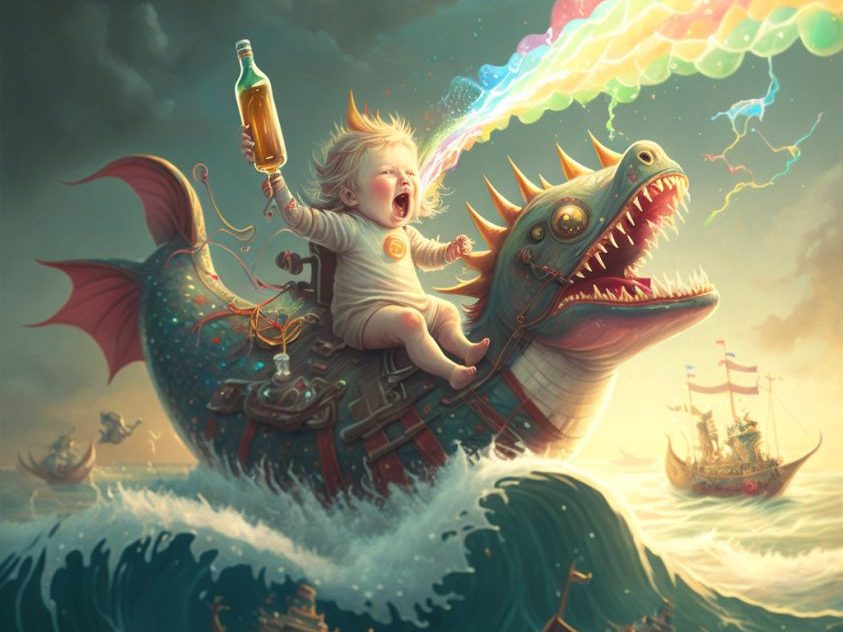
What action do the authors take? They start training a new model with 1 million tokens from the vocabulary in an unexpected way. XLM-R previously existed, however, with this upgrade, it will become XLM-V. The writers were determined to see what kind of improvement they could make with such a significant increase in tokens.
Related article: AI Model Training Costs Are Expected to Rise from $100 Million to $500 Million by 2030 |
What about XLM-V is new that XLM-R did not?
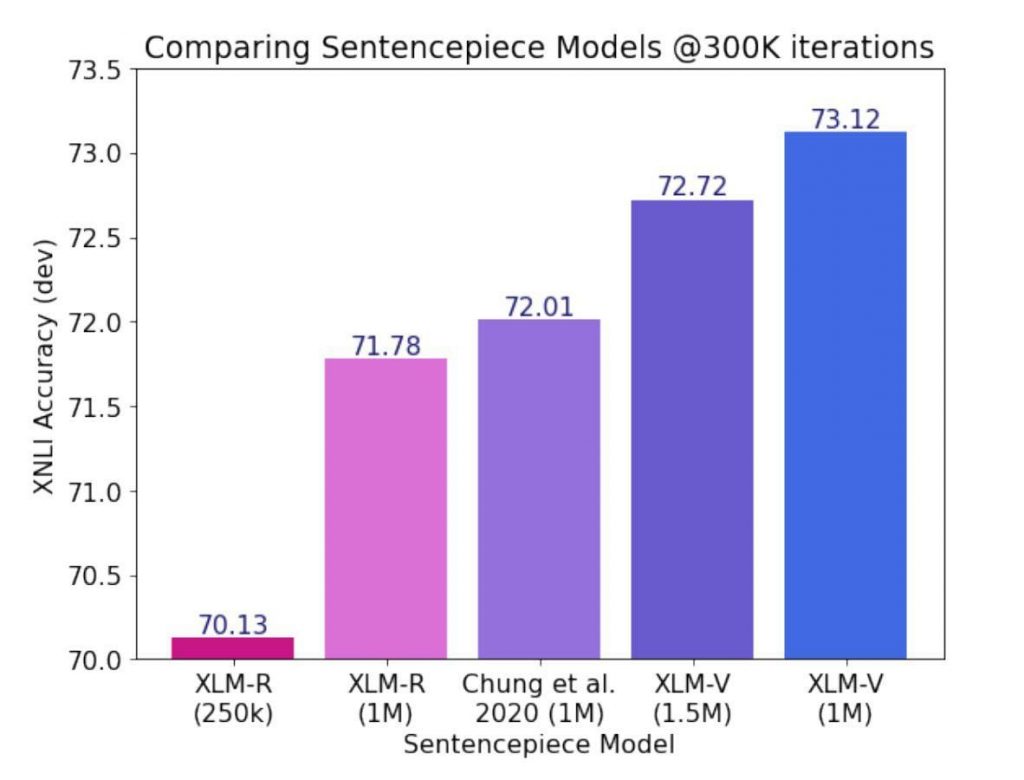
The Improving Multilingual Models with Language-Clustered Vocabularies method is used to construct lexical representation vectors for each language as follows: for each language in the set of languages, they make up a binary vector, each element of which is a specific word in the language. One indicates that the word is included in the language’s dictionary (you can view an image with a graphic description in the attachments.) However, by creating a vector utilizing the negative logarithmic probability of occurrence of each lexeme, the authors enhance how references are made.
- The vectors are grouped after that. Additionally, a sentencepiece model is trained on each particular cluster to stop the transfer of vocabulary between lexically unrelated languages.
- The ALP assesses a dictionary’s capacity to represent a specific language.
- Utilizing the algorithm for creating ULM dictionaries is the following step. which begins with a big initial dictionary and incrementally trims it down till the number of tokens is below a certain threshold for dictionary size.
Read more about AI:
Disclaimer
In line with the Trust Project guidelines, please note that the information provided on this page is not intended to be and should not be interpreted as legal, tax, investment, financial, or any other form of advice. It is important to only invest what you can afford to lose and to seek independent financial advice if you have any doubts. For further information, we suggest referring to the terms and conditions as well as the help and support pages provided by the issuer or advertiser. MetaversePost is committed to accurate, unbiased reporting, but market conditions are subject to change without notice.
About The Author
Damir is the team leader, product manager, and editor at Metaverse Post, covering topics such as AI/ML, AGI, LLMs, Metaverse, and Web3-related fields. His articles attract a massive audience of over a million users every month. He appears to be an expert with 10 years of experience in SEO and digital marketing. Damir has been mentioned in Mashable, Wired, Cointelegraph, The New Yorker, Inside.com, Entrepreneur, BeInCrypto, and other publications. He travels between the UAE, Turkey, Russia, and the CIS as a digital nomad. Damir earned a bachelor's degree in physics, which he believes has given him the critical thinking skills needed to be successful in the ever-changing landscape of the internet.
More articles

Damir is the team leader, product manager, and editor at Metaverse Post, covering topics such as AI/ML, AGI, LLMs, Metaverse, and Web3-related fields. His articles attract a massive audience of over a million users every month. He appears to be an expert with 10 years of experience in SEO and digital marketing. Damir has been mentioned in Mashable, Wired, Cointelegraph, The New Yorker, Inside.com, Entrepreneur, BeInCrypto, and other publications. He travels between the UAE, Turkey, Russia, and the CIS as a digital nomad. Damir earned a bachelor's degree in physics, which he believes has given him the critical thinking skills needed to be successful in the ever-changing landscape of the internet.