Gartner: The assessing the financial effects of data and AI teams has become critical

In Brief
The problem of assessing the financial effects of Data, AI and ML teams has become critical.
In the world of AI’s bright future, everyone only talks about the stunning effects of data analysis and what data teams can bring to the table in general. Have you seen these effects in reality: specific cash flows as a result of the implementation of data analysis projects? The answer is likely ambiguous. So Gartner discussed the problem of evaluating the effects of data teams at their leading conference on data and analytics this year.
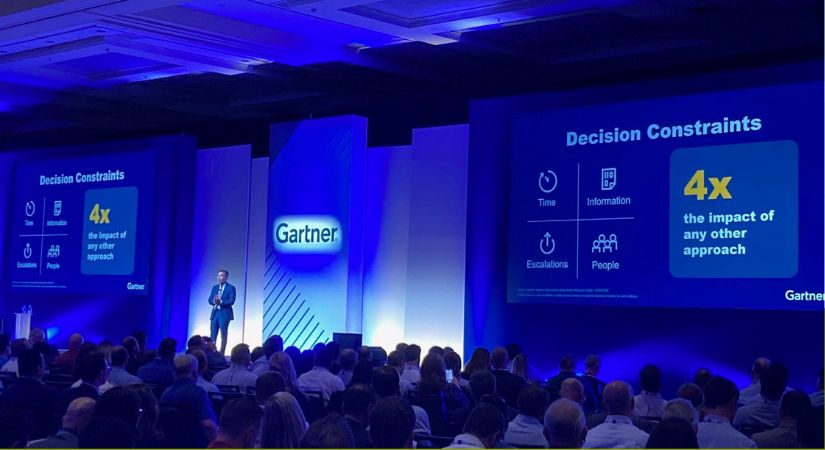
According to Gartner’s study, since 1975, there has been a steady decline in the proportion of companies that measure the specific financial impact of data analytics projects (revenue growth, cost reduction, productivity growth, and risk reduction). Already in 2020, more than 90% of investments in data (against 17% in 1975) were justified by the so-called strategic goals: the creation of innovations, data as an asset, and brand value.
And then you can talk a lot about how and why we came to this and what will happen next against the backdrop of the gathering clouds in the global macroeconomic environment.
Why has the trend formed?
Justifying the effect of data analysis in terms of strategic goals is, in many cases, quite normal. The development of the industry in recent years has already become obvious to everyone, it seems: ChatGPT here makes the last shot the last doubter. At the moment of a breakthrough, no company that wants to survive wants to remain hopelessly behind.
Justifying the effect with strategic goals is sometimes forced when you do not invest in understanding what real financial effects investments in data can bring and how this can be measured. Many companies invest huge figures in projects to improve business processes based on data, but at the same time, they save on creating a methodology for evaluating the effects of these projects (AB testing, post-investment analysis of data projects, etc.). With each new project, such companies get more and more bogged down in the trap of uncertainty; for them, the risk of the final bankruptcy of all data activity is growing, or the data team is overinflated without understanding the success of their activities.
At the same time, in practice, the introduction of such methodologies has always resulted in the greatest effects on all data projects.
What will happen next?
The dark side is the growing vulnerability of data teams in a difficult macroeconomic situation on world markets. If 90% of the effects of some types of teams cannot be “touched” because they are somewhere in the bright future, when the economic crisis intensifies, it will be these teams that will be the first to be hit. Unfortunately, the beginning of this trend was largely confirmed by 2022, and a number of large-scale layoffs in large companies.
The bright side is the increased interest in real financial impact assessments. Against the background of all of the above, we expect that in 2024–2025 there will be a trend reversal, and more investments will be justified by a real financial effect.
And this will mean an increase in interest in methods like Reliable ML: how to organize the work of data teams so that the effect of their activities is measurable and financially positive. To do this, you need to think about ML system design (in order not to get into obviously unprofitable or unrealizable projects), causal inference (in order not to fall into the trap of false patterns), and AB testing (in order to correctly understand whether your prototype will bring money when scaling).
Read more related news:
Disclaimer
In line with the Trust Project guidelines, please note that the information provided on this page is not intended to be and should not be interpreted as legal, tax, investment, financial, or any other form of advice. It is important to only invest what you can afford to lose and to seek independent financial advice if you have any doubts. For further information, we suggest referring to the terms and conditions as well as the help and support pages provided by the issuer or advertiser. MetaversePost is committed to accurate, unbiased reporting, but market conditions are subject to change without notice.
About The Author
Damir is the team leader, product manager, and editor at Metaverse Post, covering topics such as AI/ML, AGI, LLMs, Metaverse, and Web3-related fields. His articles attract a massive audience of over a million users every month. He appears to be an expert with 10 years of experience in SEO and digital marketing. Damir has been mentioned in Mashable, Wired, Cointelegraph, The New Yorker, Inside.com, Entrepreneur, BeInCrypto, and other publications. He travels between the UAE, Turkey, Russia, and the CIS as a digital nomad. Damir earned a bachelor's degree in physics, which he believes has given him the critical thinking skills needed to be successful in the ever-changing landscape of the internet.
More articles

Damir is the team leader, product manager, and editor at Metaverse Post, covering topics such as AI/ML, AGI, LLMs, Metaverse, and Web3-related fields. His articles attract a massive audience of over a million users every month. He appears to be an expert with 10 years of experience in SEO and digital marketing. Damir has been mentioned in Mashable, Wired, Cointelegraph, The New Yorker, Inside.com, Entrepreneur, BeInCrypto, and other publications. He travels between the UAE, Turkey, Russia, and the CIS as a digital nomad. Damir earned a bachelor's degree in physics, which he believes has given him the critical thinking skills needed to be successful in the ever-changing landscape of the internet.