OpenAI Unveils Prompt Engineering Guide With Six Strategies for Optimizing GPT-4 Performance


In Brief
OpenAI released its Prompt Engineering guide for GPT-4, providing detailed insights into ways to enhance the LLMs’ efficiency.
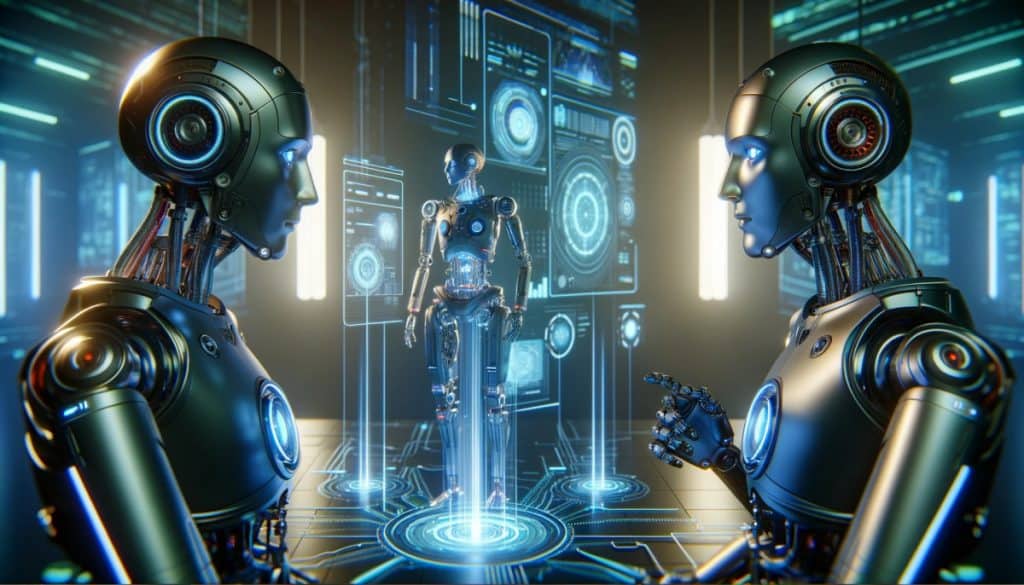
The artificial intelligence research organization OpenAI, released its Prompt Engineering guide for GPT-4. The guide offers detailed insights into optimizing the efficiency of Language Models (LLMs).
The guide outlines strategies and tactics that can be combined for greater effectiveness and includes example prompts, offering six key strategies to help users maximize the efficiency of the model.
Clear Instructions
LLM models lack intuition. If outputs are too extensive or simplistic, users should request brief or expert-level responses. The more explicit the user’s instructions, the greater the likelihood of obtaining the desired result.
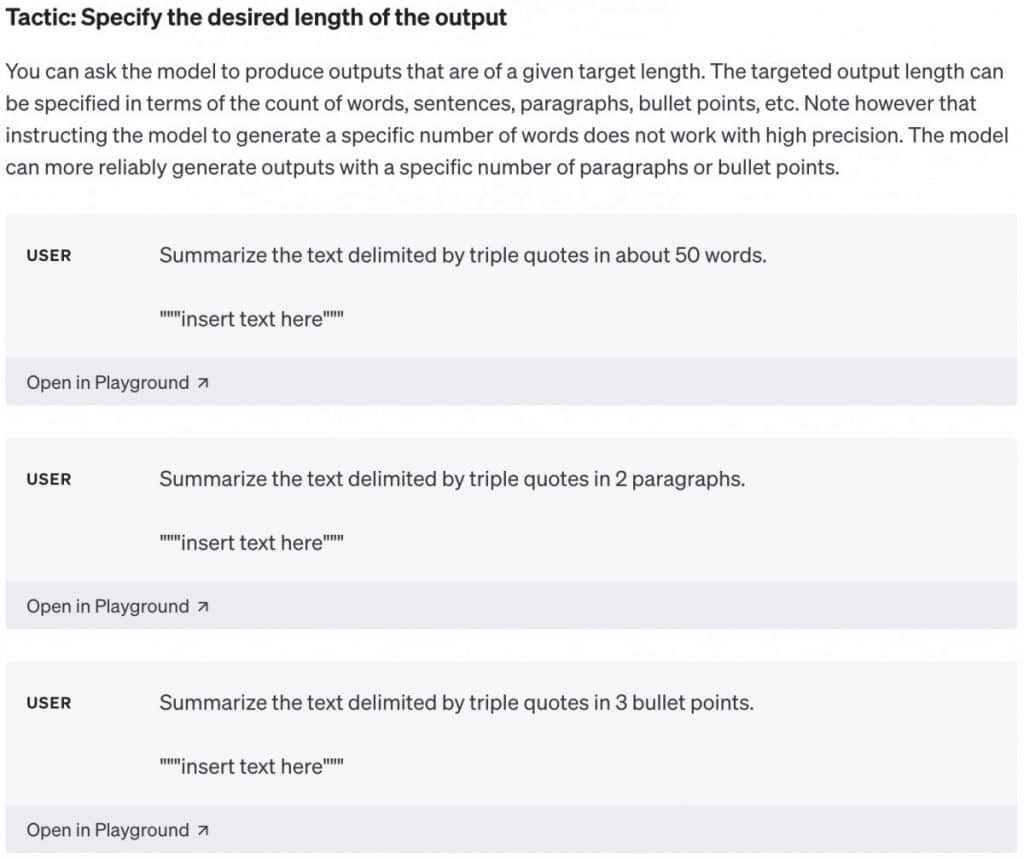
Provide Reference Texts
Language models may generate inaccurate responses, especially on obscure topics or when asked for citations and URLs. Similar to how notes assist a student, providing reference text can enhance the model’s accuracy. Users can instruct the model to answer using reference text or provide citations from it.
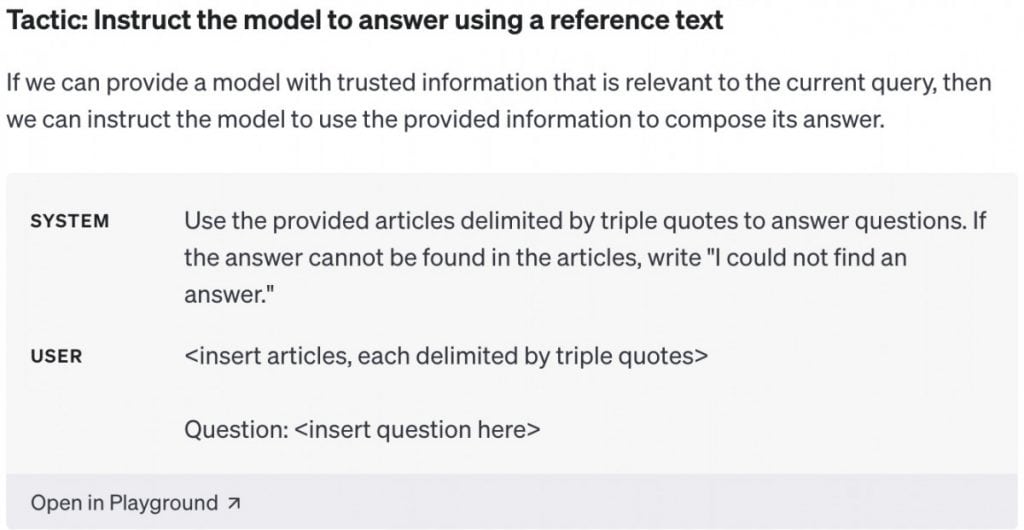
Breakdown the Complex Task into Simpler Instructions
Users should break down a complex system into modular components for improved performance. Complex tasks often have higher error rates than simpler ones. Moreover, complex tasks can be redefined as workflows of simpler tasks, where outputs from earlier tasks construct inputs for later ones.
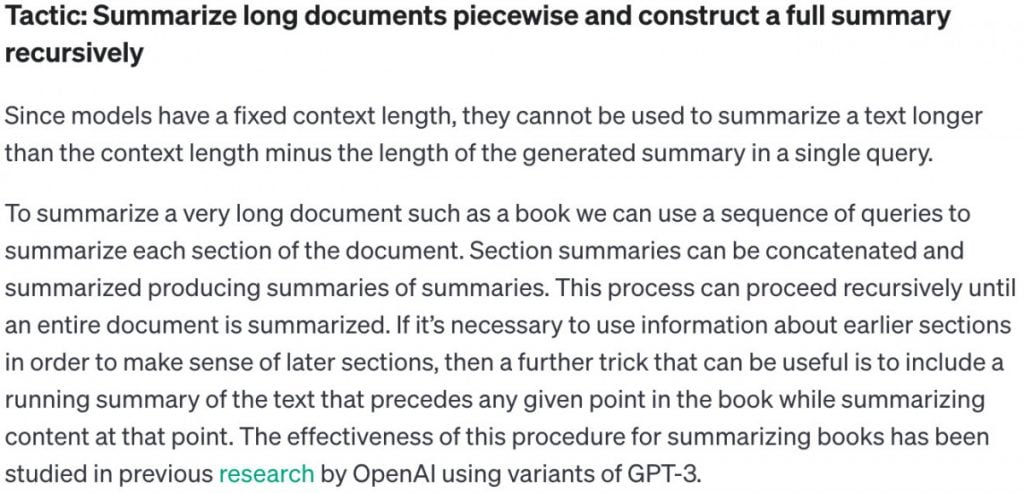
The Model Requires Time for Analysis
LLM models are more prone to reasoning errors when providing immediate responses. Requesting a “chain of thought” before receiving an answer can help the model reason its way toward more reliable and accurate responses.
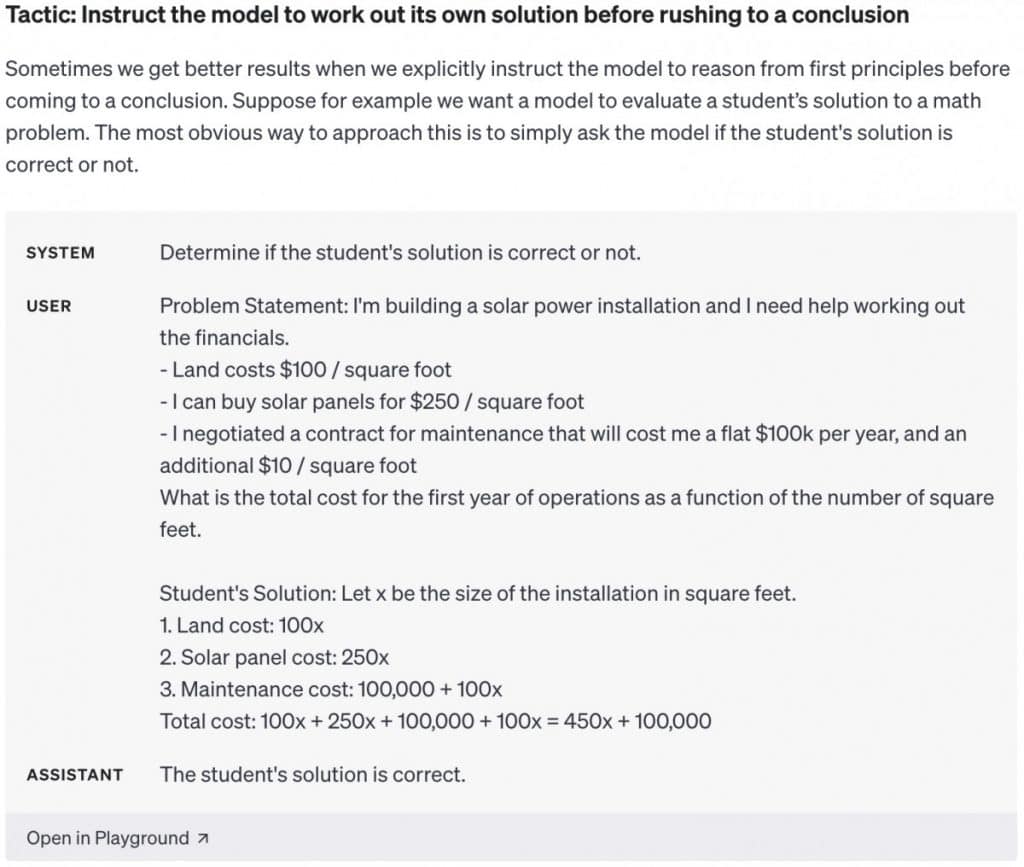
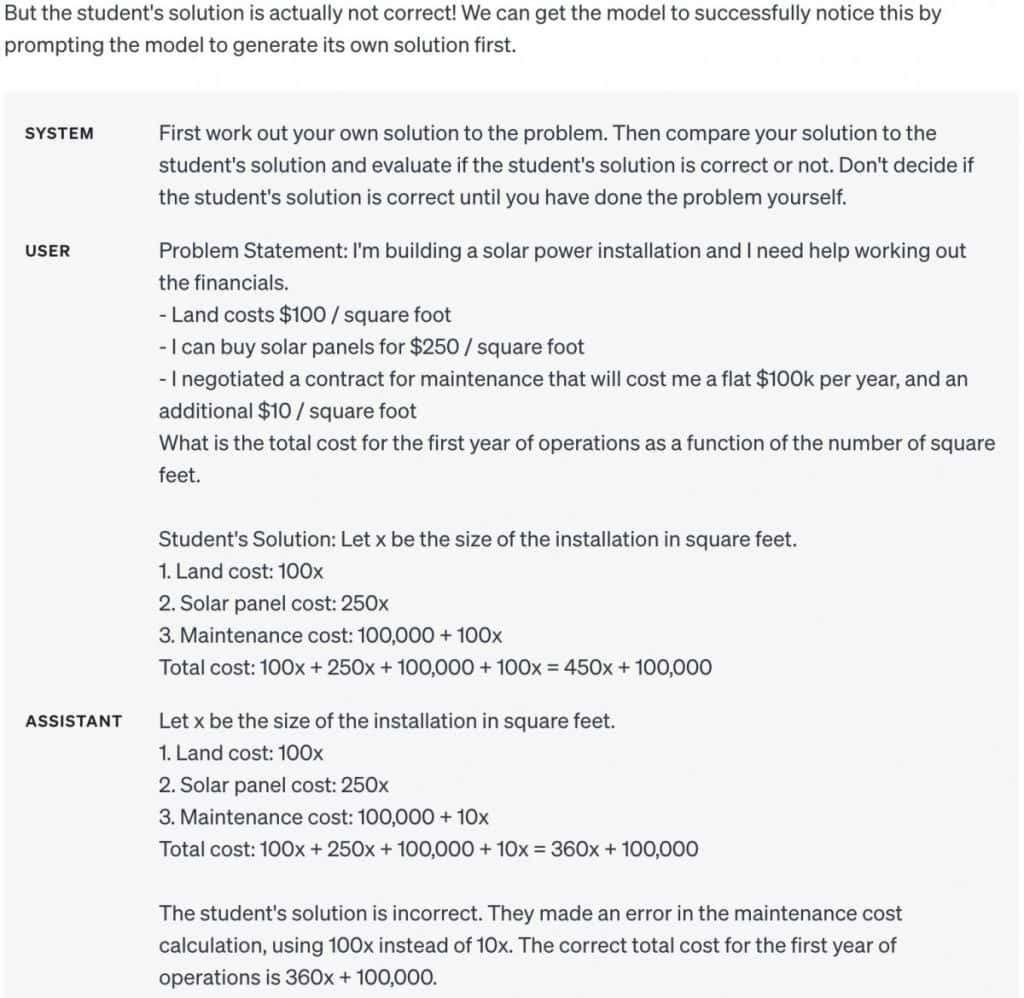
Users Should Utilize External Tools
Offset the model’s limitations by providing outputs from other tools. A code execution engine, like OpenAI’s Code Interpreter, can assist in mathematical calculations and code execution. If a task can be done more reliably or efficiently using a tool, consider offloading it for better results.
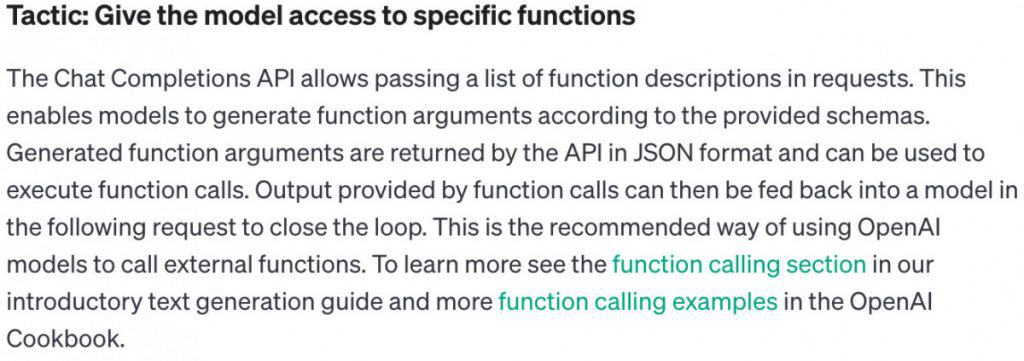
Test Changes Systematically
Enhancing performance is possible by quantifying it. While altering a prompt may improve performance in specific instances, it could lead to decreased overall performance. To ensure a change positively contributes to performance, establishing a comprehensive test suite may be essential.
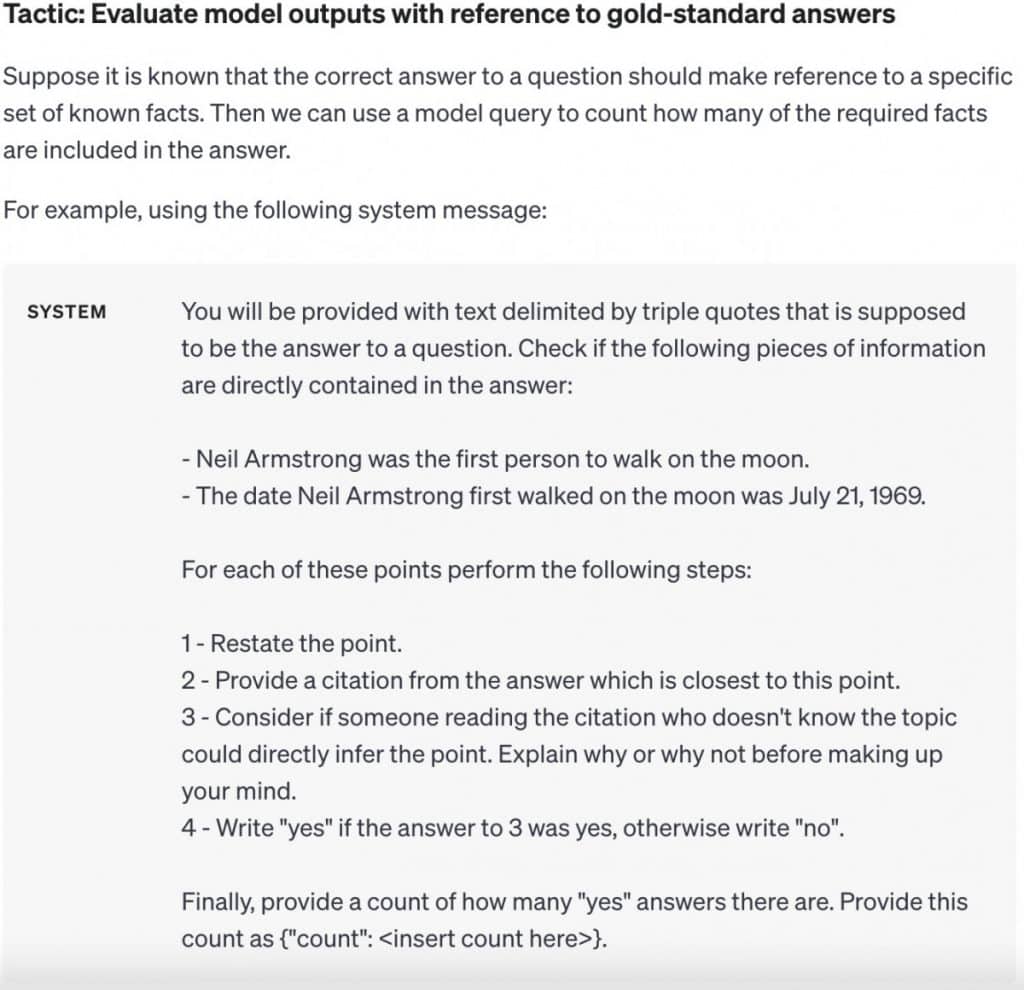
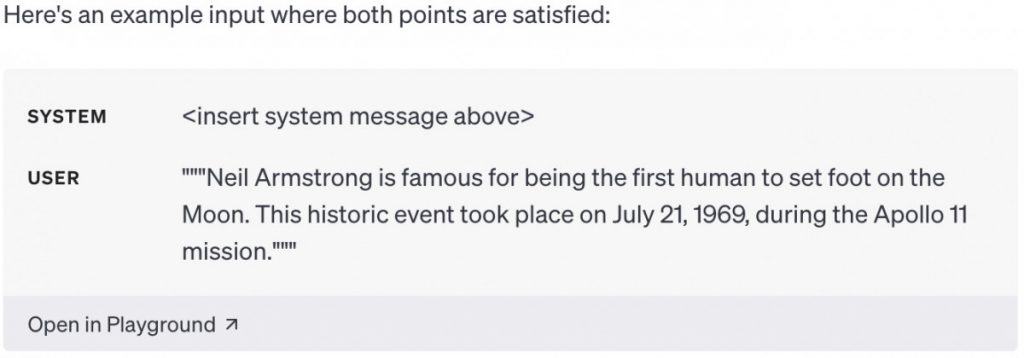
By leveraging the Prompt Engineering guide for GPT-4, users can enhance the efficiency of LLMs through explicit methods and tactics ensuring its optimal performance in diverse scenarios.
Disclaimer
In line with the Trust Project guidelines, please note that the information provided on this page is not intended to be and should not be interpreted as legal, tax, investment, financial, or any other form of advice. It is important to only invest what you can afford to lose and to seek independent financial advice if you have any doubts. For further information, we suggest referring to the terms and conditions as well as the help and support pages provided by the issuer or advertiser. MetaversePost is committed to accurate, unbiased reporting, but market conditions are subject to change without notice.About The Author
Alisa is a reporter for the Metaverse Post. She focuses on investments, AI, metaverse, and everything related to Web3. Alisa has a degree in Business of Art and expertise in Art & Tech. She has developed her passion for journalism through writing for VCs, notable crypto projects, and scientific writing. You can contact her at alisa@mpost.io
More articles

Alisa is a reporter for the Metaverse Post. She focuses on investments, AI, metaverse, and everything related to Web3. Alisa has a degree in Business of Art and expertise in Art & Tech. She has developed her passion for journalism through writing for VCs, notable crypto projects, and scientific writing. You can contact her at alisa@mpost.io