TokenFlow: New Diffusion Features for Enhanced AI Video Editing Based on Text Prompts


In Brief
TokenFlow introduces a text-driven video editing approach using a text-to-image diffusion model, allowing users to edit source videos based on specific text prompts.
This method maintains consistency within the diffusion feature space, ensuring that the output aligns with the input text prompt and retains the original video’s spatial configuration and motion dynamics.
TokenFlow’s methodology is unique and efficient, ensuring temporal consistency without requiring extensive training or adjustments.
Utilizing a text-to-image diffusion model, TokenFlow offers users the opportunity to edit source videos based on specific text prompts. The result? A refined video output that not only aligns with the input text prompt but also retains the original video’s spatial configuration and motion dynamics. This achievement is grounded in TokenFlow’s principal observation: to maintain consistency in the edited video, it’s imperative to enforce consistency within the diffusion feature space.
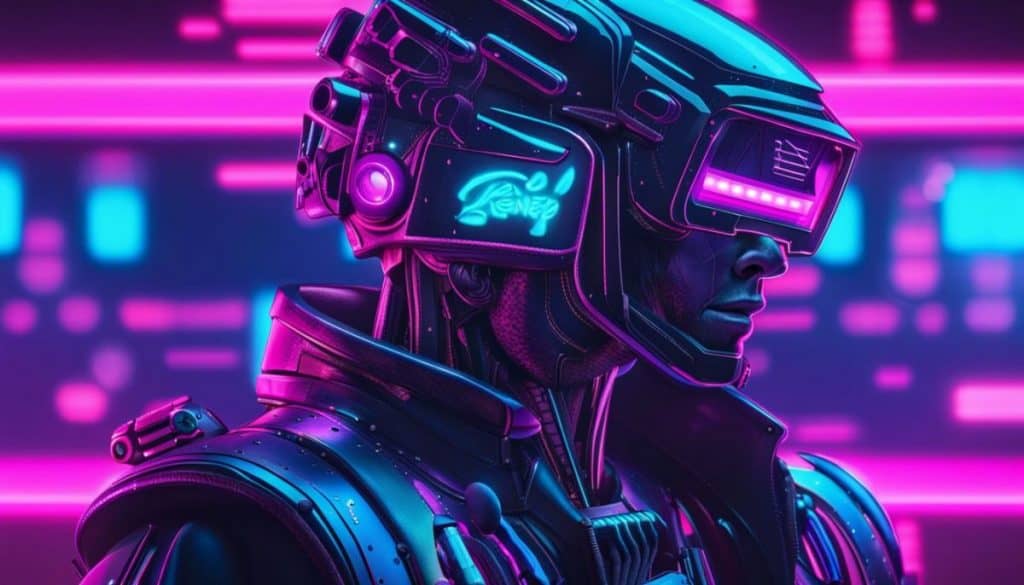
The method TokenFlow employs is both unique and efficient. Instead of relying on extensive training or adjustments, the framework leverages diffusion features derived from inter-frame correspondences inherent in the model. This capability allows TokenFlow to align seamlessly with pre-existing text-to-image editing techniques.
A deeper dive into TokenFlow’s methodology reveals its adeptness at maintaining temporal consistency. The framework observes that a video’s temporal consistency is intrinsically linked to its feature representation’s temporal consistency. Traditional methods, when editing videos frame by frame, can often disrupt this natural feature consistency. TokenFlow, however, ensures that this consistency remains unaffected.
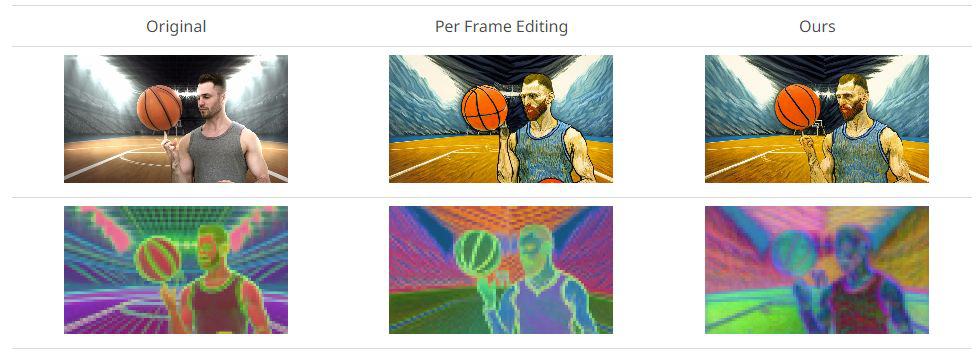
At the heart of this process is TokenFlow’s method of achieving a temporally-consistent edit. It does so by emphasizing uniformity within the internal diffusion features across different frames during the editing progression. This is facilitated by the propagation of a selected set of edited features across frames, using connections between the original video features.
The process unfolds as follows:
- For an input video, each frame is inverted to extract its tokens, essentially the output features from self-attention modules.
- Inter-frame feature correspondences are then derived using a nearest-neighbor search.
- During denoising, keyframes from the video undergo joint editing via an extended-attention block, leading to the creation of the edited tokens.
- These edited tokens are then disseminated across the video, in line with the pre-established correspondences of the original video features.
It’s noteworthy that TokenFlow’s approach comes at a time when the generative AI sector is witnessing a shift towards video. The framework, with its focus on preserving the spatial and motion aspects of input videos while ensuring consistent editing, sets a new standard. Moreover, by eliminating the need for training or fine-tuning, TokenFlow proves its adaptability and potential to work in harmony with other text-to-image editing tools. This capability has been further exemplified through TokenFlow’s superior editing outcomes on a diverse range of real-world video content.
Read more about AI:
Disclaimer
In line with the Trust Project guidelines, please note that the information provided on this page is not intended to be and should not be interpreted as legal, tax, investment, financial, or any other form of advice. It is important to only invest what you can afford to lose and to seek independent financial advice if you have any doubts. For further information, we suggest referring to the terms and conditions as well as the help and support pages provided by the issuer or advertiser. MetaversePost is committed to accurate, unbiased reporting, but market conditions are subject to change without notice.
About The Author
Damir is the team leader, product manager, and editor at Metaverse Post, covering topics such as AI/ML, AGI, LLMs, Metaverse, and Web3-related fields. His articles attract a massive audience of over a million users every month. He appears to be an expert with 10 years of experience in SEO and digital marketing. Damir has been mentioned in Mashable, Wired, Cointelegraph, The New Yorker, Inside.com, Entrepreneur, BeInCrypto, and other publications. He travels between the UAE, Turkey, Russia, and the CIS as a digital nomad. Damir earned a bachelor's degree in physics, which he believes has given him the critical thinking skills needed to be successful in the ever-changing landscape of the internet.
More articles

Damir is the team leader, product manager, and editor at Metaverse Post, covering topics such as AI/ML, AGI, LLMs, Metaverse, and Web3-related fields. His articles attract a massive audience of over a million users every month. He appears to be an expert with 10 years of experience in SEO and digital marketing. Damir has been mentioned in Mashable, Wired, Cointelegraph, The New Yorker, Inside.com, Entrepreneur, BeInCrypto, and other publications. He travels between the UAE, Turkey, Russia, and the CIS as a digital nomad. Damir earned a bachelor's degree in physics, which he believes has given him the critical thinking skills needed to be successful in the ever-changing landscape of the internet.