AI Can Detect High-Risk of Lung Cancer Among Non Smokers, says RSNA


In Brief
Researchers have developed an AI tool that can identify non-smokers who are at high risk for lung cancer using a routine chest X-ray image.
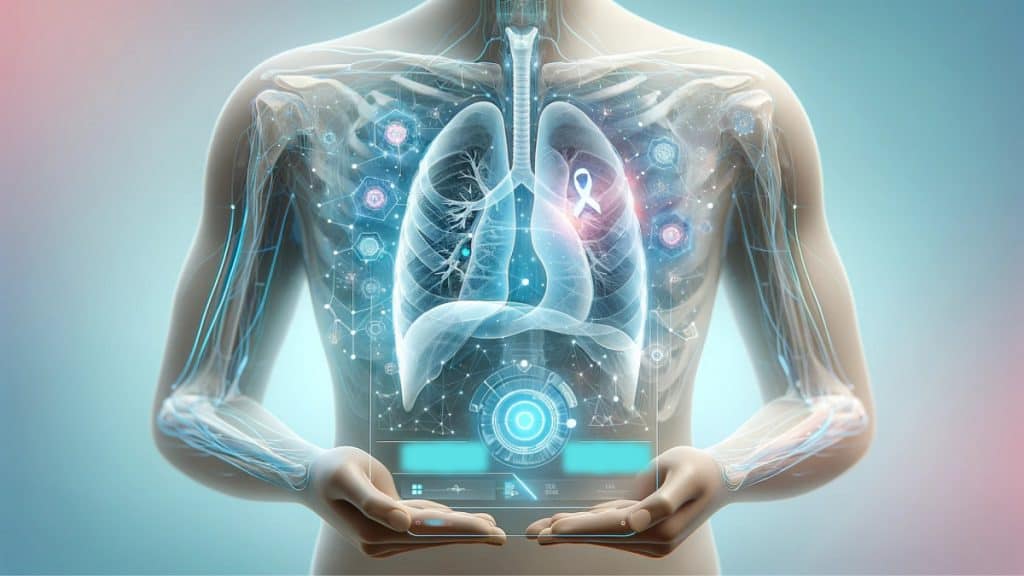
Researchers at the Cardiovascular Imaging Research Center (CIRC) have developed an AI tool that can identify non-smokers who are at high risk for lung cancer using a routine chest X-ray image.
Approximately 10-20 per cent of lung cancers occur in “never-smokers” — people who have never smoked cigarettes or smoked fewer than 100 cigarettes in their lifetime.
In this study, which is going to be presented next week at the annual meeting of the Radiological Society of North America (RSNA), researchers aim to enhance the prediction of lung cancer risk in never-smokers. They are conducting tests to determine the effectiveness of a deep learning model in identifying non-smokers with a high risk of lung cancer. The model analyzes chest X-rays extracted from electronic medical records to identify patterns associated with the disease.
Deep learning, an advanced form of artificial intelligence, is utilized to train the model to search for these patterns in X-ray images.
“A major advantage to our approach is that it only requires a single chest-X-ray image, which is one of the most common tests in medicine and widely available in the electronic medical record,” said lead author Anika S. Walia, a medical student at Boston University School of Medicine.
The “CXR-Lung-Risk” model was developed using 147,497 chest X-rays of 40,643 asymptomatic smokers and non-smokers to predict lung-related mortality risk, based on a single chest X-ray image as input.
The researchers externally validated the model in a separate group of non-smokers having routine outpatient chest X-rays from 2013 to 2014. The primary outcome was a six-year incident of lung cancer. Of 17,407 patients (mean age 63 years) included in the study, 28 per cent were deemed high risk by the deep learning model, and 2.9 per cent of these patients later had a diagnosis of lung cancer.
The high-risk group well exceeded the 1.3 per cent six-year risk threshold where lung cancer screening CT is recommended by National Comprehensive Cancer Network guidelines.
There was still a 2.1 times greater risk of developing lung cancer in the high-risk group compared to the low-risk group.
“This AI tool opens the door for opportunistic screening for non-smokers at high risk of lung cancer, using existing chest X-rays in the electronic medical record,” said Michael T. Lu, director of artificial intelligence and co-director of CIRC at Massachusetts General Hospital (MGH).
“Since cigarette smoking rates are declining, approaches to detect lung cancer early in those who do not smoke are going to be increasingly important.”
AI from MIT to Detect Early Signs of Lung Cancer
In yet another development early this year, researchers at the Mass General Cancer Center and the Massachusetts Institute of Technology (MIT) in Cambridge assert that artificial intelligence (AI) has the capability to identify early indications of the disease several years before detection through a CT scan.
They have developed an AI tool, named Sybil. According to a recent study, Sybil demonstrated remarkable accuracy, successfully predicting whether an individual would develop lung cancer in the next year with a success rate ranging from 86% to 94%.
The Centers for Disease Control and Prevention presently advocate that adults at risk for lung cancer undergo an annual low-dose CT scan as a screening measure for the disease.
However, despite regular screenings, there are limitations to what even the most skilled radiologist can detect, and this is where Sybil comes into play.
Dr. Lecia Sequist, an oncologist and the program director of the Cancer Early Detection and Diagnostics Clinic at Massachusetts General Hospital, emphasizes the limitations of the human eye, stating, “The naked eye cannot see everything.” She highlights that the AI developed, named Sybil, employs a completely different approach in analyzing the scans compared to a human radiologist.
Disclaimer
In line with the Trust Project guidelines, please note that the information provided on this page is not intended to be and should not be interpreted as legal, tax, investment, financial, or any other form of advice. It is important to only invest what you can afford to lose and to seek independent financial advice if you have any doubts. For further information, we suggest referring to the terms and conditions as well as the help and support pages provided by the issuer or advertiser. MetaversePost is committed to accurate, unbiased reporting, but market conditions are subject to change without notice.About The Author
Kumar is an experienced Tech Journalist with a specialization in the dynamic intersections of AI/ML, marketing technology, and emerging fields such as crypto, blockchain, and NFTs. With over 3 years of experience in the industry, Kumar has established a proven track record in crafting compelling narratives, conducting insightful interviews, and delivering comprehensive insights. Kumar's expertise lies in producing high-impact content, including articles, reports, and research publications for prominent industry platforms. With a unique skill set that combines technical knowledge and storytelling, Kumar excels at communicating complex technological concepts to diverse audiences in a clear and engaging manner.
More articles

Kumar is an experienced Tech Journalist with a specialization in the dynamic intersections of AI/ML, marketing technology, and emerging fields such as crypto, blockchain, and NFTs. With over 3 years of experience in the industry, Kumar has established a proven track record in crafting compelling narratives, conducting insightful interviews, and delivering comprehensive insights. Kumar's expertise lies in producing high-impact content, including articles, reports, and research publications for prominent industry platforms. With a unique skill set that combines technical knowledge and storytelling, Kumar excels at communicating complex technological concepts to diverse audiences in a clear and engaging manner.